Transforming Business Efficiency with Labeling Tool Machine Learning
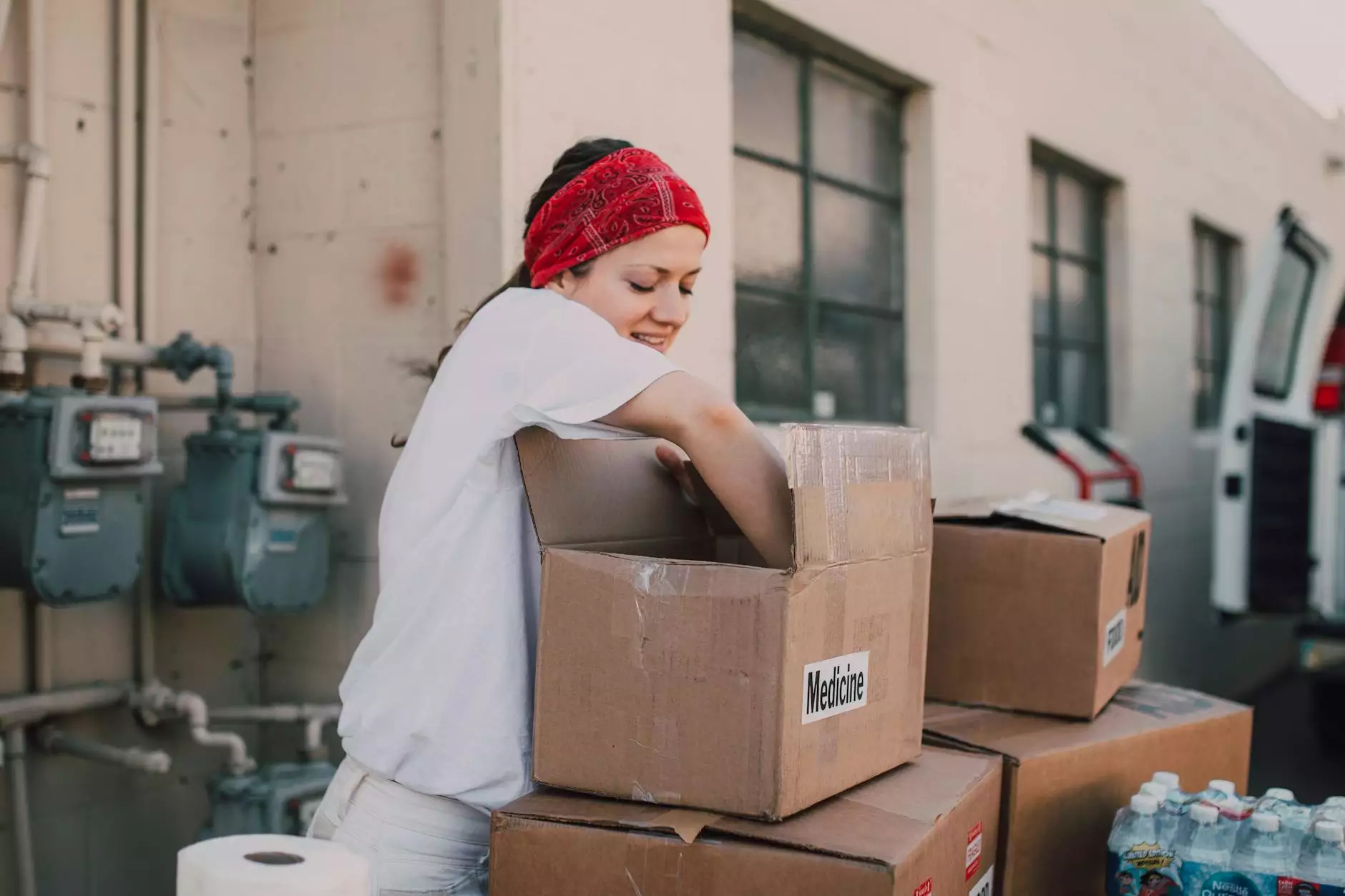
In today’s data-driven world, businesses must leverage every available tool to optimize their processes. One of the most significant advancements in this space is the use of the labeling tool machine learning, a solution that not only accelerates data annotation but also enhances the quality of machine learning models.
Understanding the Importance of Data Annotation
Data annotation is the cornerstone of machine learning as it allows algorithms to learn from high-quality labeled data. This process enables computers to understand and interpret information in ways that mimic human cognition. Here are several reasons why effective data annotation is pivotal:
- Improves Accuracy: Well-annotated data leads to better model performance.
- Increases Efficiency: Automated labeling can significantly reduce the time required for data preparation.
- Scalability: High-quality training datasets are necessary for scaling machine learning projects.
- Accessibility: Properly annotated data can be easily shared and utilized across various platforms and teams.
What is a Labeling Tool in Machine Learning?
A labeling tool in machine learning is a software application or platform that facilitates the process of annotating datasets. These tools equip data scientists, annotators, and teams with the capabilities to efficiently process large volumes of data. The integration of machine learning into labeling tools further automates and enhances this process, resulting in superior accuracy and speed.
Key Features of Machine Learning Labeling Tools
When selecting a labeling tool machine learning, it’s crucial to consider the features that can directly influence your business outcomes. Here are essential features to look for:
- Automation: Advanced algorithms can auto-generate labels, reducing the manual effort needed.
- Collaboration Tools: Features that allow teams to work together seamlessly on annotations.
- Multi-Format Support: The ability to annotate various data types, including images, text, audio, and video.
- Quality Control Systems: Mechanisms to ensure the accuracy and reliability of annotations.
Benefits of Leveraging a Data Annotation Tool
Employing a robust data annotation tool can transform your business operations in several ways:
1. Enhanced Productivity
With the integration of a labeling tool machine learning, your teams can achieve much higher productivity levels. Automation capabilities reduce repetitive tasks, allowing human annotators to focus on more complex and nuanced data.
2. Cost Efficiency
Manual annotation can be labor-intensive and costly. By utilizing a machine learning-enabled tool, you can cut down costs associated with hiring large teams of annotators. Automation helps in reallocating resources to other pivotal business operations.
3. Improved Data Quality
Machine learning algorithms can assist in ensuring higher data quality through consistent labeling standards. Human oversight combined with machine suggestions provides a balanced approach to data accuracy.
4. Faster Time to Market
With quicker annotation times, businesses can bring their machine learning projects to market faster. This agility can significantly impact competitiveness in rapidly evolving industries.
Implementing a Data Annotation Platform
A data annotation platform is an integrated suite of tools and services designed to manage the entire lifecycle of data annotation. Implementing such a platform involves several steps:
1. Define Project Scope and Objective
Before diving into the annotation process, it’s vital to clearly define the scope and objectives of your project. Understanding what you aim to achieve will guide your annotation strategies.
2. Select the Right Tools
Your choice of a labeling tool machine learning should align with your specific needs. Different projects may require different capabilities, so evaluate tools based on functionality and scalability.
3. Train Your Annotators
Even with automated tools, human annotators play a crucial role in quality assurance. Provide training to help them understand the nuances of your specific requirements and the capabilities of the tool.
4. Monitor and Evaluate
Regular monitoring and evaluation of the annotation process ensure that quality standards are met. Feedback loops between annotators, data scientists, and project managers can drive continuous improvement.
Challenges in Data Annotation and How to Overcome Them
While data annotation tools and platforms offer remarkable advantages, they are not without challenges. Understanding these challenges and devising strategies to overcome them is crucial for successful implementation:
1. Data Complexity
Complex datasets often require sophisticated labeling that can be challenging to automate completely. Combining automated tools with expert annotators often yields the best results.
2. Ensuring Consistency
Inconsistent annotations can compromise data quality. Establishing clear guidelines and checkpoints can help maintain consistency across the board.
3. Scalability Issues
As your business grows, so will your data needs. Choose a labeling tool that scales well with your operations to avoid bottlenecks in your data processes.
Conclusion: The Future of Business Leveraging Data Annotation
The integration of labeling tool machine learning into business processes is not just a trend; it represents the future of data handling and machine learning implementation. By optimizing data annotation practices, businesses can lead the charge in innovation, improve operational efficiencies, and ultimately deliver better products and services to their clients.
As you consider your data annotation strategies, look to KeyLabs.ai for expert insights and advanced tools that can help you navigate this crucial aspect of machine learning. The possibilities are endless when you harness the power of effective data annotation.